Increasing data quality by interpolating missing data in cow monitoring
Predicting and estimating gaps in dairy cow dataResearcher Keni Ren and her colleagues have identified an accurate method for interpolating missing data in systems for monitoring dairy cows in the barn. This knowledge can be used to improve the indoor positioning systems used by modern farms and researchers to study cows’ activities.
Cows that are allowed to roam freely indoor, in a barn, spend their time resting, walking, standing, feeding and visiting the milking robot. Their individual behavioral patterns and social activities are important for cow welfare and for avoiding disease outbreaks.
Real-time systems for monitoring animals, e. g. ultra-wideband (UWB) technology, are increasingly used but not perfectly accurate. Almost always, there will be a lot of time-gaps in this kind of studies, where data are missing due to physical structures in the barn, calibration problems or communication failures between the tags carried by the cows and the receiver anchors in the locating system.
In the field of statistics, there are a number of interpolation methods used to improve the quality of data sets, by predicting and estimating the unknown data. Keni Ren and her colleagues decided to find out which interpolation method would be the most accurate one to use when studying the positioning data for dairy cows inside a free-stall dairy farm.
We investigated four different types of methods. All four are well known methods but we didn’t know how much they differ or which one to prefer in this specific application, with real cow life, says Keni Ren.
By monitoring dairy cows, researchers and farmers get to know more about how the animals behave and interact. The knowledge can be used to improve livestock farming in terms of animal welfare and health.
The modified Akima method was the best
During six days, 69 cows were observed in detail to analyse where the missing data happened and how long the gaps lasted. The data from the 20 most reliable tags were compared with the interpolations - of simulated missing data - from the four different interpolation methods (1) previous position, 2) linear interpolation, 3) cubic spline data interpolation and 4) modified Akima interpolation).
The modified Akima interpolation method showed the lowest error distance for all investigated cow activities; walking, feeding, resting and standing. In other words it had the highest prediction accuracy for the various activities in the barn, says Keni Ren.
Cows walk a little, they stop, turn around, and walk in another direction. Sometimes they take a longer distance walk in the barn, but they spend a lot of time just resting. In the feeding area the cows often move back and forth within a limited area. The algorithm in the modified Akima interpolation method is designed to avoid overshoots without being too flattened, and this fits the various types of movements in different areas of the barn.
The advantage of using the Akima method, compared to the other methods, is the greatest when it comes to filling in the gaps of missing data occurring when the animal is walking. I was surprised by how the error distance was stabilized after just one minute of missing data, using this method.
Researchers often mention the problems with missing data, when they publish their studies on animal behavior.
The different interpolation methods are frequently used, but I have not seen anyone verifying which method is the best one for studying the social network of dairy cattle before, says Keni Ren.
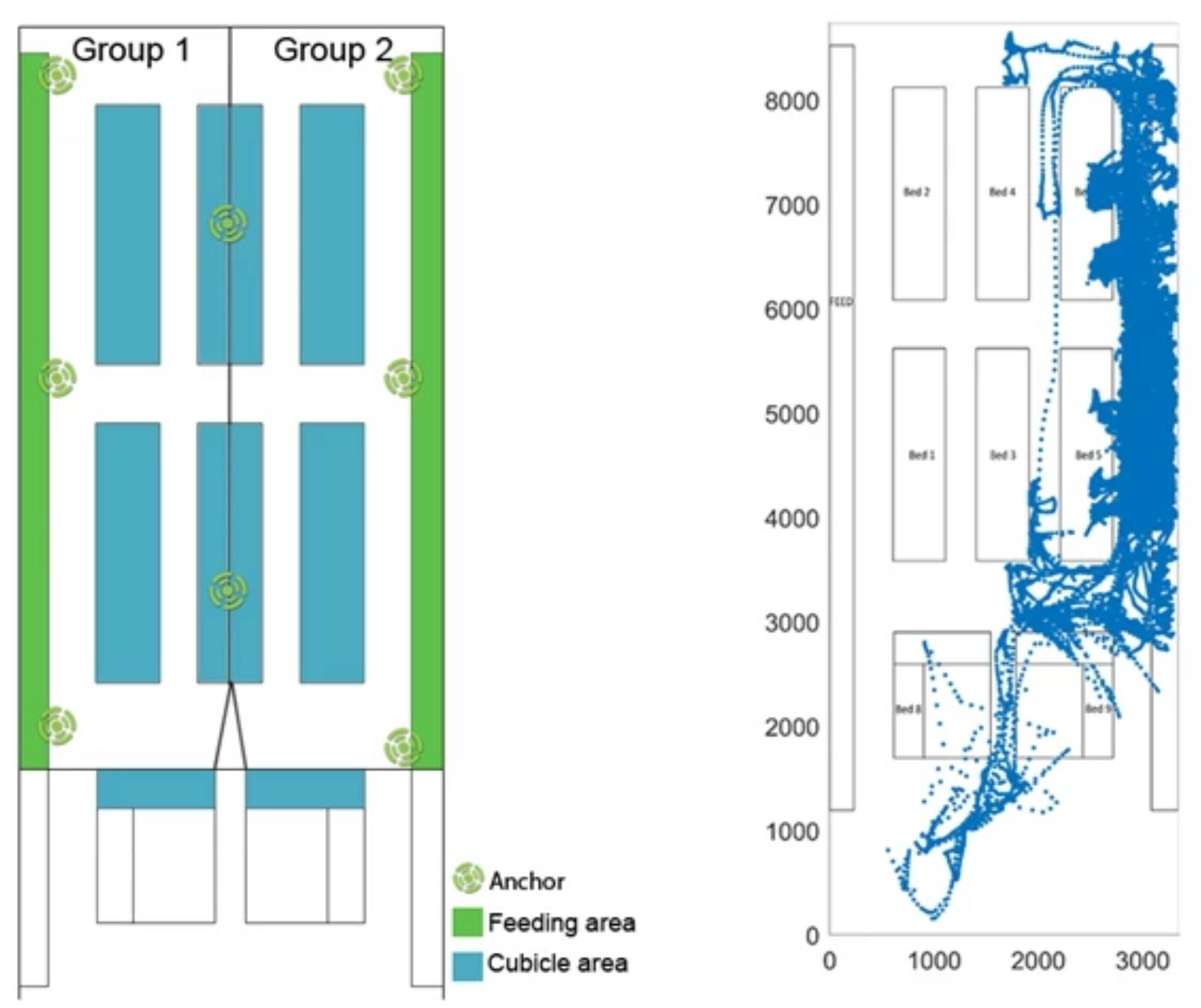